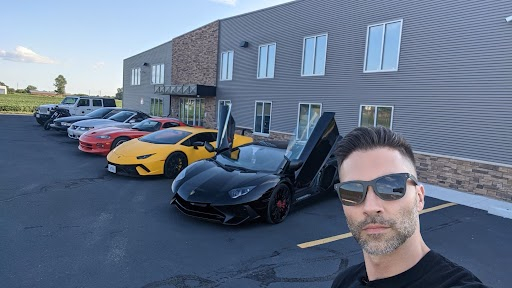
Dan Crosby
My site offers real-world insights on entrepreneurship, business growth, and personal development, with direct mentorship opportunities to help you achieve your own success.
By registering you agree to Substack's Terms of Service, our Privacy Policy, and our Information Collection Notice